Introduction
Artificial Intelligence (AI) is a dynamic and complex field, steadily redefining the healthcare sector. The broad spectrum of AI technologies is bringing a paradigm shift in patient care, medical research, and hospital operations. As AI continues to evolve and transform, it becomes critical for healthcare professionals, administrators, and stakeholders to understand the diverse forms of AI, their capabilities, and their relevance in the healthcare context.
Demystifying AI in Healthcare
Before diving into the types of AI, it’s essential to unravel what AI means in the healthcare realm. Artificial Intelligence in healthcare refers to the use of complex algorithms and software, also known as ‘intelligent agents,’ to emulate human cognition in the analysis, interpretation, and comprehension of complicated medical and healthcare data. The primary aim of health-related AI applications is to analyze relationships between prevention or treatment techniques and patient outcomes.
Machine Learning in Healthcare: Predictive Care and Personalized Treatment
Machine learning, a subset of AI, involves training a computer to learn, adapt, and make data-driven decisions. It allows healthcare systems to create more precise and efficient algorithms. The potential applications of machine learning in healthcare are immense. It is instrumental in predicting disease progression, analyzing genetic information for personalized treatments, and managing electronic health records.
Predictive algorithms in machine learning can process vast quantities of data and extract patterns that may be invisible to human analysis. These algorithms can predict disease outcomes, helping healthcare providers take preventive measures. For instance, machine learning models are being used to predict sepsis, a life-threatening reaction to infection that can cause organ failure. Early prediction gives healthcare providers crucial time to intervene and improve patient outcomes.
The advent of genomics and personalized medicine has been greatly facilitated by machine learning. AI models can analyze large-scale genomic data and identify genetic markers associated with disease, enabling the development of individualized treatments.
Moreover, machine learning can significantly enhance the handling of electronic health records. Algorithms can be trained to read unstructured patient notes, extract critical information, predict patient trajectories, and suggest personalized treatment plans.
Natural Language Processing in Healthcare: Unstructured Data Analysis
Natural Language Processing (NLP) focuses on understanding and interpreting human language. In healthcare, it is a powerful tool for extracting useful insights from unstructured data. NLP can analyze clinical notes, research articles, patient feedback, and social media posts to derive meaningful information.
In the realm of public health, NLP can be used to track disease outbreaks or public health crises by analyzing social media posts or news articles. For instance, researchers have successfully used NLP techniques to track the spread of diseases like influenza and COVID-19.
NLP can also assist clinicians in extracting useful information from patient records, which often contain unstructured notes filled with complex medical jargon. NLP tools can convert these notes into structured data that can be easily analyzed and used for patient care.
Moreover, patient experience can be significantly improved using NLP. Sentiment analysis, an application of NLP, can help healthcare providers understand patient feedback and improve their services accordingly. For example, if a majority of patient reviews mention long waiting times or unavailability of appointments, healthcare providers can work to resolve these issues and enhance patient satisfaction.
Deep Learning in Healthcare: Radiology and Pathology
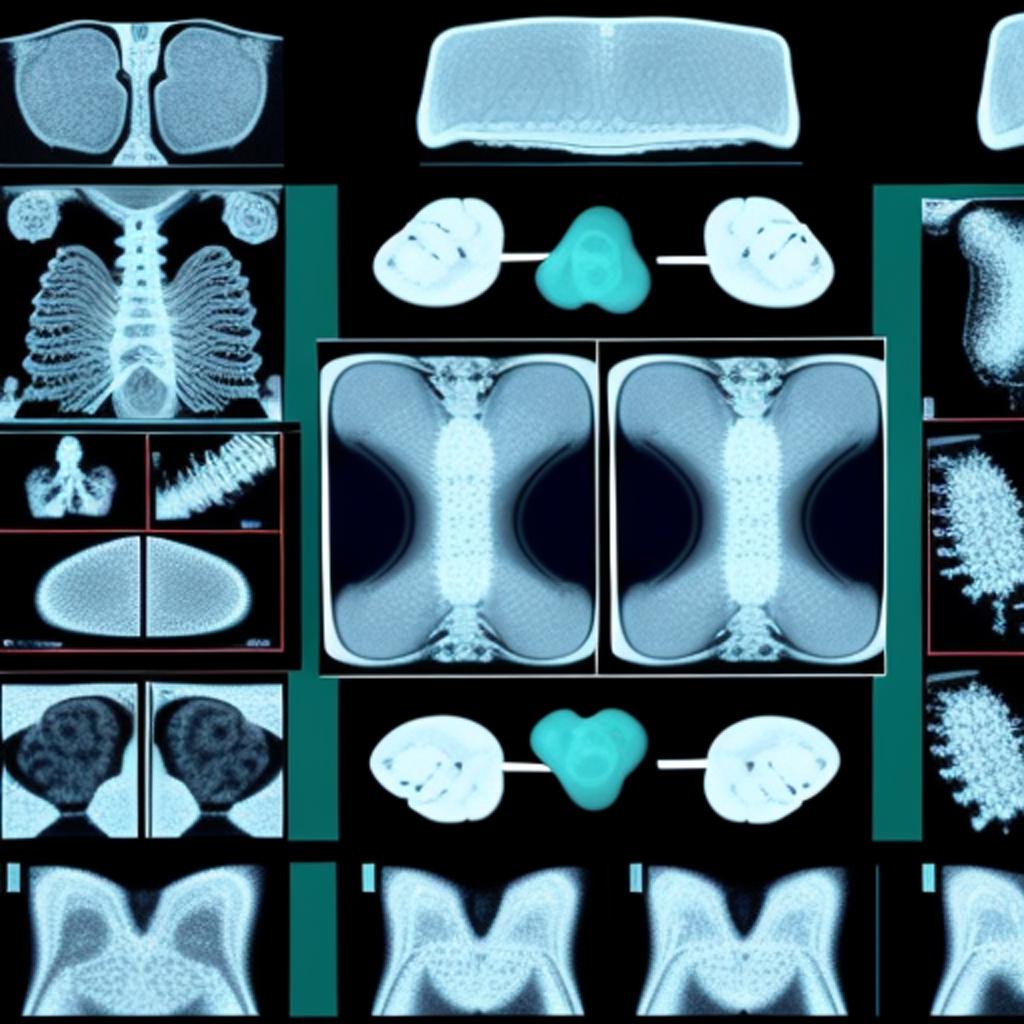
Deep learning, a type of machine learning, uses multi-layered neural networks to analyze data. It has shown great promise in image-heavy medical disciplines like radiology and pathology. Deep learning models can analyze radiology images to detect signs of disease. In some cases, they have been found to perform at par with trained radiologists.
For example, deep learning algorithms can detect lung nodules in chest CT scans, aiding in early scans, aiding in early diagnosis of lung cancer. They can also analyze mammograms for early detection of breast cancer or assess brain scans to identify signs of stroke, brain injury, or tumors. By providing rapid and accurate analyses of complex images, deep learning can help radiologists improve their productivity and diagnostic accuracy.
In pathology, deep learning can revolutionize the way microscopic images are analyzed. Traditional microscopic image analysis can be time-consuming and subject to human error. However, deep learning algorithms can swiftly analyze these images, identify abnormalities, and even classify different types of cells, diseases, or stages of disease progression.
For instance, AI algorithms have been trained to detect and classify skin cancers, enabling early and accurate diagnosis. Similar applications exist in the detection of diseases such as prostate cancer, where AI can accurately analyze tissue samples to aid in diagnosis.
Deep learning also has promising applications in the realm of ophthalmology. AI algorithms can analyze retinal images to diagnose conditions like diabetic retinopathy and age-related macular degeneration. These technologies not only increase diagnostic accuracy but also reduce the workload for overburdened healthcare professionals, allowing them to focus more on patient care.
Robotic Process Automation in Healthcare: Streamlining Administrative Processes
Robotic Process Automation (RPA), another subset of AI, refers to software robots or ‘bots’ that can automate routine tasks. In healthcare, RPA has the potential to streamline a myriad of administrative processes, such as appointment scheduling, billing, and claims processing.
RPA can help healthcare providers automate patient scheduling, ensuring that appointments are made efficiently and at times that work best for both the patient and provider. By automating this process, healthcare staff can save time and focus on more critical tasks.
When it comes to billing and claims processing, RPA can greatly enhance efficiency and accuracy. RPA bots can swiftly process billing information, reduce errors, and expedite claim settlements. These bots can also flag problematic claims, allowing healthcare staff to intervene and rectify issues promptly.
Conclusion
As AI continues to evolve, it brings an array of potential benefits to the healthcare sector, ranging from improved patient care and outcomes to enhanced operational efficiency. By understanding the different types of AI and their applications in healthcare, professionals in the sector can make informed decisions about adopting these technologies and effectively leveraging them to improve healthcare delivery.
It is important to note that while AI offers enormous potential, its implementation in healthcare should be done judiciously and ethically, always prioritizing patient safety, privacy, and the enhancement of human care.
At Propelen, we are committed to helping healthcare organizations navigate this journey, offering expert guidance, tailored solutions, and ongoing support. We understand that every healthcare organization is unique, and we strive to provide AI solutions that meet your unique needs and circumstances. We invite you to reach out to us today!
This is the fifth in a series of blog posts about the emergence of Artificial Intelligence in healthcare, written by Propelen LLC CEO Sokhona Sillah. See all articles in this series: ArtificlaI Intelligence and Healthcare